About The Workshop
Real-world Human-Robot Interaction (HRI) requires robots to adeptly perceive and understand the dynamic human-centred environments in which they operate. Recent decades have seen remarkable advancements that have endowed robots with exceptional perception capabilities. However, much of this progress is grounded in pattern recognition and statistical correlation-based machine learning (ML), neglecting the intrinsic structures and interdependencies between variables in observational data and the underlying causal relationships that govern the emergence of these dependencies. Causality precisely focuses on unraveling such causal structures and relationships inherent in the data. Many challenges within ML and HRI, including generalisation and bias issues, can be attributed to this ignorance of cause-and-effect relationships between data variables. The first workshop on “Causal-HRI: Causal Learning for Human-Robot Interaction” aims to bring together research perspectives from Causal Discovery and Inference and Causal Learning, in general, to real-world HRI applications. The objective of this workshop is to explore strategies that can not only embed robots with capabilities to discover cause-and-effect relationships from observations, allowing them to generalise to unseen interaction settings, but also to enable users to understand robot behaviours, moving beyond the ‘black-box’ models used by these robots. This workshop aims to facilitate an exchange of views through invited keynote presentations, contributed talks, group discussions and poster sessions, encouraging collaborations across diverse scientific communities. The theme of HRI 2024, “HRI in the real world”, will inform the overarching theme of this workshop, encouraging discussions on HRI theories, methods, designs and studies focussed on leveraging Causal Learning for enhancing real-world HRI.
Join the workshop Slack Channel to stay updated and connect with the rest of the community.
Call for Papers
We invite authors to submit their contributions as 3-4 page papers (plus additional pages for references and appendices), highlighting their experimental results, technical reports and case studies discussing Causal Learning for Human-Robot Interaction. In particular, we will encourage submissions addressing the theme of HRI 2024: ``HRI in the real-world''. All submissions will be peer-reviewed for their novelty, relevance, contribution to the field and technical soundness.
Similar to the main HRI conference, all submissions should be made following the general ACM SIG format (“sigconf”, double column format) and not the SIGCHI format. Templates can be found here or on overleaf directly. Accepted papers will be published on the workshop website. Based on authors’ consent and preferences, the proceedings of the workshop will be compiled as a single submission or as an indexed compendium of individual papers and made available on arXiv.
Call for Posters
We also invite researchers to submit position articles as 1-2 page extended abstracts for poster presentations. These abstracts will receive a light review that examines fit and factual correctness, but the authors will receive feedback from the audience during the workshop.
All submissions to be made via EasyChair.
The call for papers and posters can also be found here.
Please contact lguerdan [AT] cs.cmu.edu for any queries regarding submission.
Topics
Topics of interest include, but are not limited to:
- Causal inference
- Counterfactual reasoning for robotics
- Causal representation learning
- Causal Learning for Skill-discovery for Robots
- Causal discovery of latent graphs for robotic behavioural learning
- Explanations for Robot Behaviours
- Generalised representation learning for Human-Robot Interaction
- Scene understanding with Causal Inference
- Causal Learning for Human behaviour understanding
- Causal Learning for State/Action-space inferences
- Explainable Human-Robot Interaction
- Applications for Causal HRI
- Research datasets, software, open-source tools, hardware analysis, system benchmarks in/for Causal HRI
Important Dates
- Paper Submission Deadline:
02 February, 202409 February, 2024 - Notification of Acceptance (Papers):
16 February, 2024 - Poster Submission Deadline:
16 February, 2024 - Notification of Acceptance (Posters):
23 February, 2024 - Camera-ready Deadline:
01 March, 2024 04 March, 2024(All deadlines are at 23:59 Anywhere on Earth time) - Workshop Day: 11 March, 2024
Keynote Speakers
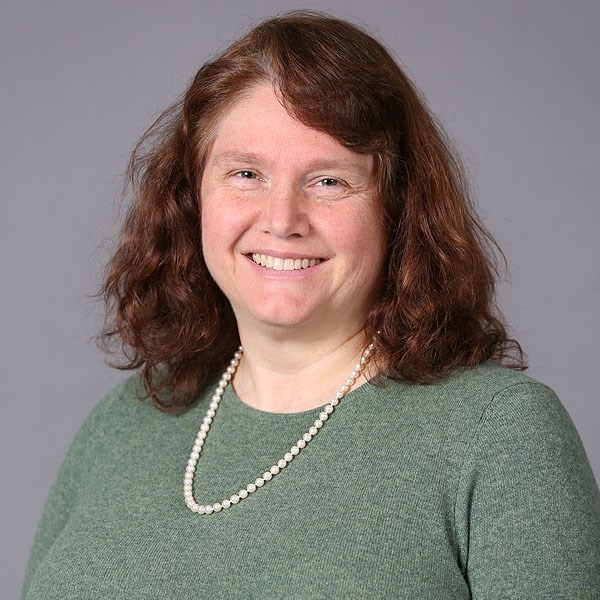
Prof Holly Yanco
University of Massachusetts Lowell
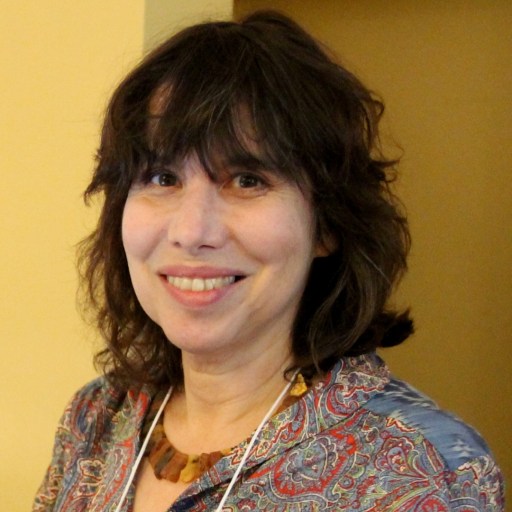
Prof Alison Gopnik
University of California at Berkeley
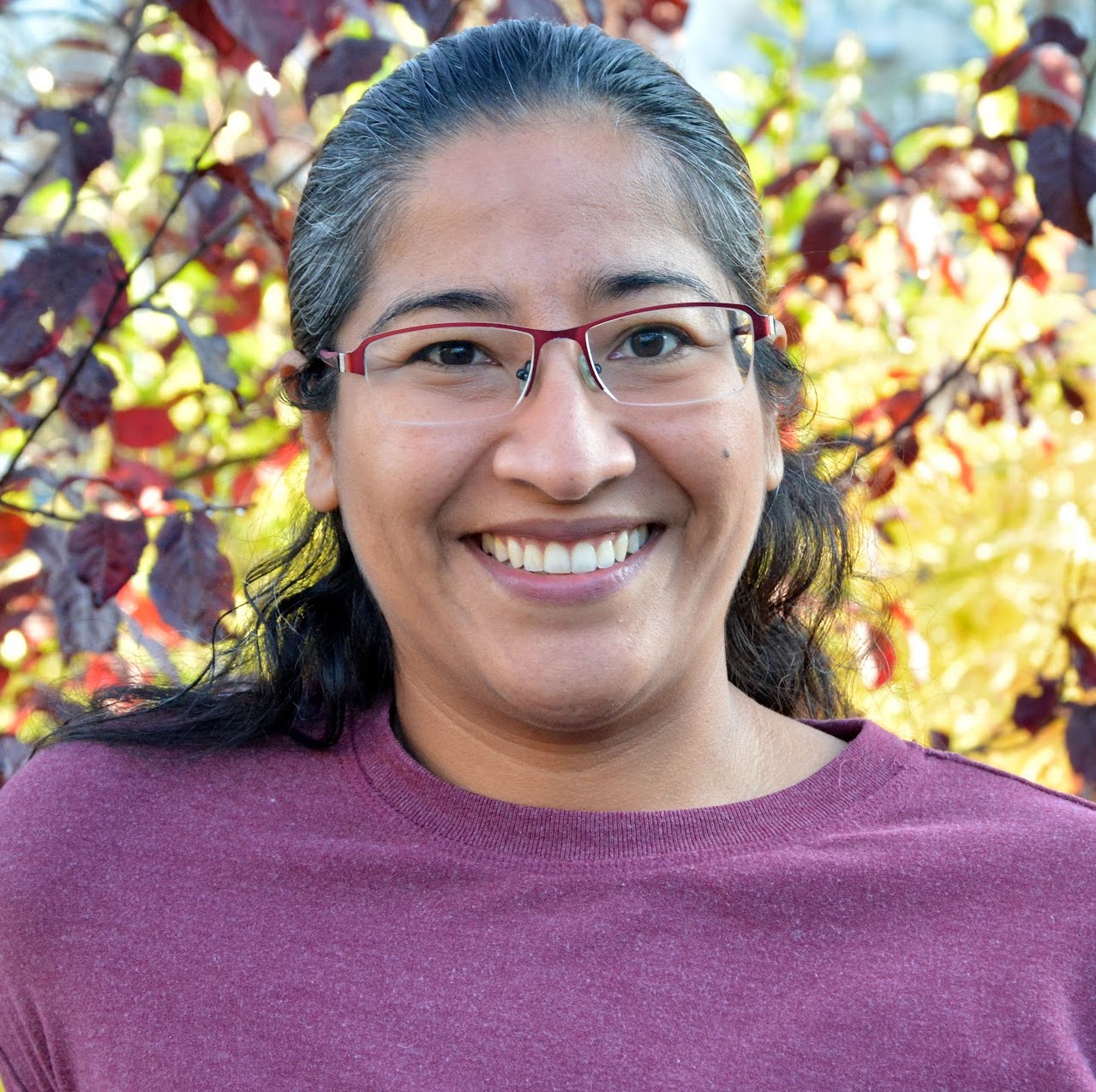
Prof Karinne Ramirez-Amaro
Chalmers University of Technology
Workshop Schedule
Opening and Introduction
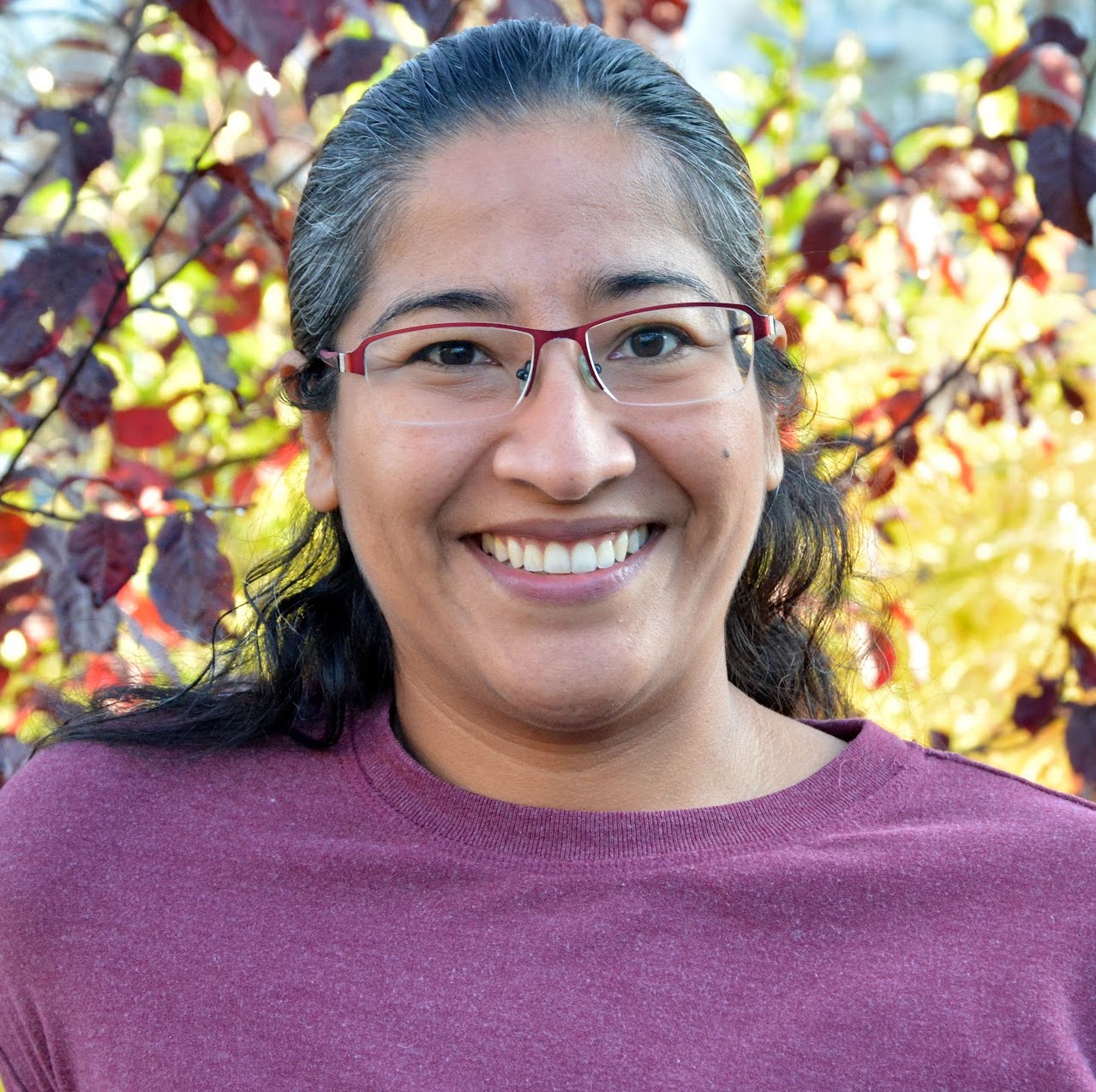
Keynote Prof Karinne Ramirez-Amaro
Title: Explainable AI meets Robotics - Robots that Learn Causal-based models to predict and prevent failures.
Abstract: The advances in Collaborative Robots (Cobots) have rapidly increased with the development of novel data- and knowledge-driven methods. These methods allow robots, to some extent, to explain their decisions. This research area is known as Explainable AI and is gaining importance in the robotics community. One advantage of such methods is the increase of human trust towards Cobots since robots can explain their decisions, especially when errors occur or when facing new situations. Explainability is a challenging and important component when deploying Cobots into real and dynamic environments. In this talk, I will introduce our proposed causal-based approach to empower robots with rapid prediction and prevention of both immediate and future failures. I will explain our efforts to automatically learn the cause-effect relationships of tasks, and the generation of explanations for unsuccessful actions. Our goal is to enable robots to understand “why” things happen, which will allow them to learn from their mistakes, thus improving their future performances. Therefore, the new generation of Cobots should reason based on past experiences while providing explanations in case of errors. Thus, improving the autonomy of robots and humans’ trust to work with robots.
Keynote Prof Alison Gopnik
Title: Empowerment as causal learning, causal learning as empowerment.
Abstract: Cognitive scientists have applied advances in our formal understanding of causation in philosophy and computer science, particularly within the Causal Bayes Net formalism, to understand human causal learning. In parallel, in the very different tradition of reinforcement learning, researchers have developed the idea of an intrinsic reward signal called “empowerment”. An agent is rewarded for maximizing the mutual information between its actions and their outcomes, regardless of the external reward value of those outcomes. In other words, the agent is rewarded if variation in an action systematically leads to parallel variation in an outcome so that the value of the action predicts the value of the outcome. The result is an agent that has maximal control over its environment. We argue that “empowerment” may be an important bridge between classical Bayesian causal learning and reinforcement learning and may help to characterize causal learning in humans and enable it in machines. Empowerment may explain distinctive empirical features of children’s causal learning, as well as providing a more tractable computational account of how that learning is possible. More strongly we make the philosophical argument that causal learning and empowerment gain are very closely related. In particular, our hypothesis is that if an agent learns an accurate causal model of the world they will necessarily increase their empowerment, and, vice versa, increasing empowerment will lead to a more accurate (if implicit) causal model of the world. This has implications for both cognitive science accounts of causal learning and for the metaphysics of causation.
Coffee Break
Contributed Talks
- ROS-Causal: A ROS-based Causal Analysis Framework for Human-Robot Interaction Applications [paper]
Luca Castri, Gloria Beraldo, Sariah Mghames, Marc Hanheide, and Nicola Bellotto - A Human-Centered Approach for Bootstrapping Causal Graph Creation [paper]
Minh Q. Tram, Nolan B. Gutierrez, and William J. Beksi - Human causal reasoning guided autonomous object assembly under uncertainty [paper]
Semanti Basu, Semir Tatlidil, Moon Hwan Kim, Steven Sloman, and R. Iris Bahar - Causal Influence Detection for Human Robot Interaction [paper]
Yi-Shiuan Tung, Himanshu Gupta, Wei Jiang, Bradley Hayes, and Alessandro Roncone
Keynote: Prof Holly Yanco
Title: The Impact of Causal Learning on the Design and Evaluation of Human-Robot Interaction.
Abstract: All robots interact with people in some way. Robots navigating in difficult and dynamic environments often need assistance from human operators or supervisors, either in the form of teleoperation or interventions when the robot's autonomy is not able to handle the current situation. Even in more controlled environments, such as office buildings and manufacturing floors, robots may need help from people. As robots become more autonomous and ubiquitous, people will become bystanders to the systems, with little knowledge of the robot’s intent. As we develop robots that have the ability to explain their actions, how will the design and evaluation of human-robot interaction change? This talk will cover examples from a wide variety of applications, ranging from assistive technology to telepresence to exoskeletons to humanoid robots.
Group Discussion
Closing Remarks
Organisers
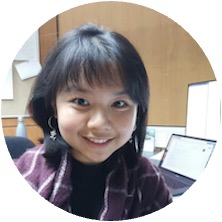
Jiaee Cheong
University of Cambridge
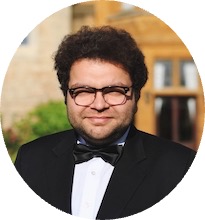
Nikhil Churamani
University of Cambridge
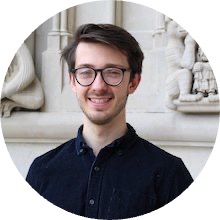
Luke Guerdan
Carnegie Mellon University
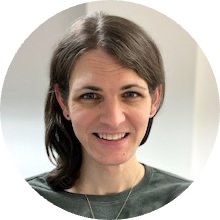
Tabitha Edith Lee
Carnegie Mellon University
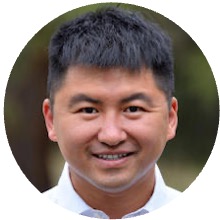
Zhao Han
University of South Florida
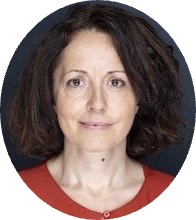
Hatice Gunes
University of Cambridge